Continuing playing with Reddit data, I thought that it might be fun to extract
discussed topics from subreddits. My idea was: get comments from a
subreddit, extract ngrams, calculate counts of ngrams, normalize counts, and
subtract them from normalized counts of ngrams from a neutral set of comments.
Small-scale
For proving the idea on a smaller scale, I’ve fetched titles, texts and
the first three levels of comments from top 1000 r/all posts (full code available in a gist),
as it should have a lot of texts from different subreddits:
get_subreddit_df('all').head()
|
id |
subreddit |
post_id |
kind |
text |
created |
score |
0 |
7mjw12_title |
all |
7mjw12 |
title |
My cab driver tonight was so excited to share ... |
1.514459e+09 |
307861 |
1 |
7mjw12_selftext |
all |
7mjw12 |
selftext |
|
1.514459e+09 |
307861 |
2 |
7mjw12_comment_druihai |
all |
7mjw12 |
comment |
I want to make good humored inappropriate joke... |
1.514460e+09 |
18336 |
3 |
7mjw12_comment_drulrp0 |
all |
7mjw12 |
comment |
Me too! It came out of nowhere- he was pretty ... |
1.514464e+09 |
8853 |
4 |
7mjw12_comment_druluji |
all |
7mjw12 |
comment |
Well, you got him to the top of Reddit, litera... |
1.514464e+09 |
4749 |
Lemmatized texts, get all 1-3 words ngrams and counted them:
df = get_tokens_df(subreddit) # Full code is in gist
df.head()
|
token |
amount |
0 |
cab |
84 |
1 |
driver |
1165 |
2 |
tonight |
360 |
3 |
excited |
245 |
4 |
share |
1793 |
Then I’ve normalized counts:
df['amount_norm'] = (df.amount - df.amount.mean()) / (df.amount.max() - df.amount.min())
df.head()
|
token |
amount |
amount_norm |
0 |
automate |
493 |
0.043316 |
1 |
boring |
108 |
0.009353 |
2 |
stuff |
1158 |
0.101979 |
3 |
python |
11177 |
0.985800 |
4 |
tinder |
29 |
0.002384 |
And as the last step, I’ve calculated diff and got top 5 ngrams with texts from
top 1000 posts from some random subreddits. Seems to be working for r/linux:
diff_tokens(tokens_dfs['linux'], tokens_dfs['all']).head()
|
token |
amount_diff |
amount_norm_diff |
5807 |
kde |
3060.0 |
1.082134 |
2543 |
debian |
1820.0 |
1.048817 |
48794 |
coc |
1058.0 |
1.028343 |
9962 |
systemd |
925.0 |
1.024769 |
11588 |
gentoo |
878.0 |
1.023506 |
Also looks ok on r/personalfinance:
diff_tokens(tokens_dfs['personalfinance'], tokens_dfs['all']).head()
|
token |
amount_diff |
amount_norm_diff |
78063 |
vanguard |
1513.0 |
1.017727 |
18396 |
etf |
1035.0 |
1.012113 |
119206 |
checking account |
732.0 |
1.008555 |
60873 |
direct deposit |
690.0 |
1.008061 |
200917 |
joint account |
679.0 |
1.007932 |
And kind of funny with r/drunk:
diff_tokens(tokens_dfs['drunk'], tokens_dfs['all']).head()
|
token |
amount_diff |
amount_norm_diff |
515158 |
honk honk honk |
144.0 |
1.019149 |
41088 |
pbr |
130.0 |
1.017247 |
49701 |
mo dog |
129.0 |
1.017112 |
93763 |
cheap beer |
74.0 |
1.009641 |
124756 |
birthday dude |
61.0 |
1.007875 |
Seems to be working on this scale.
A bit larger scale
As the next iteration, I’ve decided to try the idea on
three months of comments, which I was able to download as dumps
from pushift.io.
Shaping the data
And it’s kind of a lot of data, even compressed:
$ du -sh raw_data/*
11G raw_data/RC_2018-08.xz
10G raw_data/RC_2018-09.xz
11G raw_data/RC_2018-10.xz
Pandas basically doesn’t work on that scale, and unfortunately, I don’t
have a personal Hadoop cluster. So I’ve reinvented a wheel a bit:
graph LR
A[Reddit comments]-->B[Reddit comments wiht ngrams]
B-->C[Ngrams partitioned by subreddit and day]
C-->D[Counted partitioned ngrams]
The raw data is stored in line-delimited JSON, like:
$ xzcat raw_data/RC_2018-10.xz | head -n 2
{"archived":false,"author":"TistedLogic","author_created_utc":1312615878,"author_flair_background_color":null,"author_flair_css_class":null,"author_flair_richtext":[],"author_flair_template_id":null,"author_flair_text":null,"author_flair_text_color":null,"author_flair_type":"text","author_fullname":"t2_5mk6v","author_patreon_flair":false,"body":"Is it still r\/BoneAppleTea worthy if it's the opposite?","can_gild":true,"can_mod_post":false,"collapsed":false,"collapsed_reason":null,"controversiality":0,"created_utc":1538352000,"distinguished":null,"edited":false,"gilded":0,"gildings":{"gid_1":0,"gid_2":0,"gid_3":0},"id":"e6xucdd","is_submitter":false,"link_id":"t3_9ka1hp","no_follow":true,"parent_id":"t1_e6xu13x","permalink":"\/r\/Unexpected\/comments\/9ka1hp\/jesus_fking_woah\/e6xucdd\/","removal_reason":null,"retrieved_on":1539714091,"score":2,"send_replies":true,"stickied":false,"subreddit":"Unexpected","subreddit_id":"t5_2w67q","subreddit_name_prefixed":"r\/Unexpected","subreddit_type":"public"}
{"archived":false,"author":"misssaladfingers","author_created_utc":1536864574,"author_flair_background_color":null,"author_flair_css_class":null,"author_flair_richtext":[],"author_flair_template_id":null,"author_flair_text":null,"author_flair_text_color":null,"author_flair_type":"text","author_fullname":"t2_27d914lh","author_patreon_flair":false,"body":"I've tried and it's hit and miss. When it's good I feel more rested even though I've not slept well but sometimes it doesn't work","can_gild":true,"can_mod_post":false,"collapsed":false,"collapsed_reason":null,"controversiality":0,"created_utc":1538352000,"distinguished":null,"edited":false,"gilded":0,"gildings":{"gid_1":0,"gid_2":0,"gid_3":0},"id":"e6xucde","is_submitter":false,"link_id":"t3_9k8bp4","no_follow":true,"parent_id":"t1_e6xu9sk","permalink":"\/r\/insomnia\/comments\/9k8bp4\/melatonin\/e6xucde\/","removal_reason":null,"retrieved_on":1539714091,"score":1,"send_replies":true,"stickied":false,"subreddit":"insomnia","subreddit_id":"t5_2qh3g","subreddit_name_prefixed":"r\/insomnia","subreddit_type":"public"}
The first script add_ngrams.py
reads lines of raw data from stdin,
adds 1-3 lemmatized ngrams and writes lines in JSON to stdout.
As the amount of data is huge, I’ve gzipped the output. It took around an
hour to process month worth of comments on 12 CPU machine. Spawning more processes didn’t help as thw whole thing is quite CPU intense.
$ xzcat raw_data/RC_2018-10.xz | python3.7 add_ngrams.py | gzip > with_ngrams/2018-10.gz
$ zcat with_ngrams/2018-10.gz | head -n 2
{"archived": false, "author": "TistedLogic", "author_created_utc": 1312615878, "author_flair_background_color": null, "author_flair_css_class": null, "author_flair_richtext": [], "author_flair_template_id": null, "author_flair_text": null, "author_flair_text_color": null, "author_flair_type": "text", "author_fullname": "t2_5mk6v", "author_patreon_flair": false, "body": "Is it still r/BoneAppleTea worthy if it's the opposite?", "can_gild": true, "can_mod_post": false, "collapsed": false, "collapsed_reason": null, "controversiality": 0, "created_utc": 1538352000, "distinguished": null, "edited": false, "gilded": 0, "gildings": {"gid_1": 0, "gid_2": 0, "gid_3": 0}, "id": "e6xucdd", "is_submitter": false, "link_id": "t3_9ka1hp", "no_follow": true, "parent_id": "t1_e6xu13x", "permalink": "/r/Unexpected/comments/9ka1hp/jesus_fking_woah/e6xucdd/", "removal_reason": null, "retrieved_on": 1539714091, "score": 2, "send_replies": true, "stickied": false, "subreddit": "Unexpected", "subreddit_id": "t5_2w67q", "subreddit_name_prefixed": "r/Unexpected", "subreddit_type": "public", "ngrams": ["still", "r/boneappletea", "worthy", "'s", "opposite", "still r/boneappletea", "r/boneappletea worthy", "worthy 's", "'s opposite", "still r/boneappletea worthy", "r/boneappletea worthy 's", "worthy 's opposite"]}
{"archived": false, "author": "1-2-3RightMeow", "author_created_utc": 1515801270, "author_flair_background_color": null, "author_flair_css_class": null, "author_flair_richtext": [], "author_flair_template_id": null, "author_flair_text": null, "author_flair_text_color": null, "author_flair_type": "text", "author_fullname": "t2_rrwodxc", "author_patreon_flair": false, "body": "Nice! I\u2019m going out for dinner with him right and I\u2019ll check when I get home. I\u2019m very interested to read that", "can_gild": true, "can_mod_post": false, "collapsed": false, "collapsed_reason": null, "controversiality": 0, "created_utc": 1538352000, "distinguished": null, "edited": false, "gilded": 0, "gildings": {"gid_1": 0, "gid_2": 0, "gid_3": 0}, "id": "e6xucdp", "is_submitter": true, "link_id": "t3_9k9x6m", "no_follow": false, "parent_id": "t1_e6xsm3n", "permalink": "/r/Glitch_in_the_Matrix/comments/9k9x6m/my_boyfriend_and_i_lost_10_hours/e6xucdp/", "removal_reason": null, "retrieved_on": 1539714092, "score": 42, "send_replies": true, "stickied": false, "subreddit": "Glitch_in_the_Matrix", "subreddit_id": "t5_2tcwa", "subreddit_name_prefixed": "r/Glitch_in_the_Matrix", "subreddit_type": "public", "ngrams": ["nice", "go", "dinner", "right", "check", "get", "home", "interested", "read", "nice go", "go dinner", "dinner right", "right check", "check get", "get home", "home interested", "interested read", "nice go dinner", "go dinner right", "dinner right check", "right check get", "check get home", "get home interested", "home interested read"]}
The next script partition.py
reads stdin and writes files like
2018-10-10_AskReddit
with just ngrams to a folder passed as an
argument.
$ zcat with_ngrams/2018-10.gz | python3.7 parition.py partitions
$ cat partitions/2018-10-10_AskReddit | head -n 5
"gt"
"money"
"go"
"administration"
"building"
For three months of comments it created a lot of files:
$ ls partitions | wc -l
2715472
After that I’ve counted ngrams in partitions with group_count.py
:
$ python3.7 group_count.py partitions counted
$ cat counted/2018-10-10_AskReddit | head -n 5
["gt", 7010]
["money", 3648]
["go", 25812]
["administration", 108]
["building", 573]
As r/all isn’t a real subreddit and it’s not possible to get it from the dump,
I’ve chosen r/AskReddit as a source of “neutral” ngrams, for that I’ve
calculated the aggregated count of ngrams with aggreage_whole.py
:
$ python3.7 aggreage_whole.py AskReddit > aggregated/askreddit_whole.json
$ cat aggregated/askreddit_whole.json | head -n 5
[["trick", 26691], ["people", 1638951], ["take", 844834], ["zammy", 10], ["wine", 17315], ["trick people", 515], ["people take", 10336], ["take zammy", 2], ["zammy wine", 2], ["trick people take", 4], ["people take zammy", 2]...
Playing with the data
First of all, I’ve read “neutral” ngrams, removed ngrams appeared
less than 100 times as otherwise it wasn’t fitting in RAM
and calculated normalized count:
whole_askreddit_df = pd.read_json('aggregated/askreddit_whole.json', orient='values')
whole_askreddit_df = whole_askreddit_df.rename(columns={0: 'ngram', 1: 'amount'})
whole_askreddit_df = whole_askreddit_df[whole_askreddit_df.amount > 99]
whole_askreddit_df['amount_norm'] = norm(whole_askreddit_df.amount)
|
ngram |
amount |
amount_norm |
0 |
trick |
26691 |
0.008026 |
1 |
people |
1638951 |
0.492943 |
2 |
take |
844834 |
0.254098 |
4 |
wine |
17315 |
0.005206 |
5 |
trick people |
515 |
0.000153 |
To be sure that the idea is still valid, I’ve randomly checked
r/television for 10th October:
television_10_10_df = pd \
.read_json('counted/2018-10-10_television', lines=True) \
.rename(columns={0: 'ngram', 1: 'amount'})
television_10_10_df['amount_norm'] = norm(television_10_10_df.amount)
television_10_10_df = television_10_10_df.merge(whole_askreddit_df, how='left', on='ngram', suffixes=('_left', '_right'))
television_10_10_df['diff'] = television_10_10_df.amount_norm_left - television_10_10_df.amount_norm_right
television_10_10_df \
.sort_values('diff', ascending=False) \
.head()
|
ngram |
amount_left |
amount_norm_left |
amount_right |
amount_norm_right |
diff |
13 |
show |
1299 |
0.699950 |
319715.0 |
0.096158 |
0.603792 |
32 |
season |
963 |
0.518525 |
65229.0 |
0.019617 |
0.498908 |
19 |
character |
514 |
0.276084 |
101931.0 |
0.030656 |
0.245428 |
4 |
episode |
408 |
0.218849 |
81729.0 |
0.024580 |
0.194269 |
35 |
watch |
534 |
0.286883 |
320204.0 |
0.096306 |
0.190578 |
And just for fun, limiting to trigrams:
television_10_10_df\
[television_10_10_df.ngram.str.count(' ') >= 2] \
.sort_values('diff', ascending=False) \
.head()
|
ngram |
amount_left |
amount_norm_left |
amount_right |
amount_norm_right |
diff |
11615 |
better call saul |
15 |
0.006646 |
1033.0 |
0.000309 |
0.006337 |
36287 |
would make sense |
11 |
0.004486 |
2098.0 |
0.000629 |
0.003857 |
7242 |
ca n't wait |
12 |
0.005026 |
5396.0 |
0.001621 |
0.003405 |
86021 |
innocent proven guilty |
9 |
0.003406 |
1106.0 |
0.000331 |
0.003075 |
151 |
watch first episode |
8 |
0.002866 |
463.0 |
0.000137 |
0.002728 |
Seems to be ok, as the next step I’ve decided to get top 50 discussed topics for every available day:
r_television_by_day = diff_n_by_day( # in the gist
50, whole_askreddit_df, 'television', '2018-08-01', '2018-10-31',
exclude=['r/television'],
)
r_television_by_day[r_television_by_day.date == "2018-10-05"].head()
|
ngram |
amount_left |
amount_norm_left |
amount_right |
amount_norm_right |
diff |
date |
3 |
show |
906 |
0.725002 |
319715.0 |
0.096158 |
0.628844 |
2018-10-05 |
8 |
season |
549 |
0.438485 |
65229.0 |
0.019617 |
0.418868 |
2018-10-05 |
249 |
character |
334 |
0.265933 |
101931.0 |
0.030656 |
0.235277 |
2018-10-05 |
1635 |
episode |
322 |
0.256302 |
81729.0 |
0.024580 |
0.231723 |
2018-10-05 |
418 |
watch |
402 |
0.320508 |
320204.0 |
0.096306 |
0.224202 |
2018-10-05 |
Then I thought that it might be fun to get overall top topics
from daily top topics and make a weekly heatmap with seaborn:
r_television_by_day_top_topics = r_television_by_day \
.groupby('ngram') \
.sum()['diff'] \
.reset_index() \
.sort_values('diff', ascending=False)
r_television_by_day_top_topics.head()
|
ngram |
diff |
916 |
show |
57.649622 |
887 |
season |
37.241199 |
352 |
episode |
22.752369 |
1077 |
watch |
21.202295 |
207 |
character |
15.599798 |
r_television_only_top_df = r_television_by_day \
[['date', 'ngram', 'diff']] \
[r_television_by_day.ngram.isin(r_television_by_day_top_topics.ngram.head(10))] \
.groupby([pd.Grouper(key='date', freq='W-MON'), 'ngram']) \
.mean() \
.reset_index() \
.sort_values('date')
pivot = r_television_only_top_df \
.pivot(index='ngram', columns='date', values='diff') \
.fillna(-1)
sns.heatmap(pivot, xticklabels=r_television_only_top_df.date.dt.week.unique())
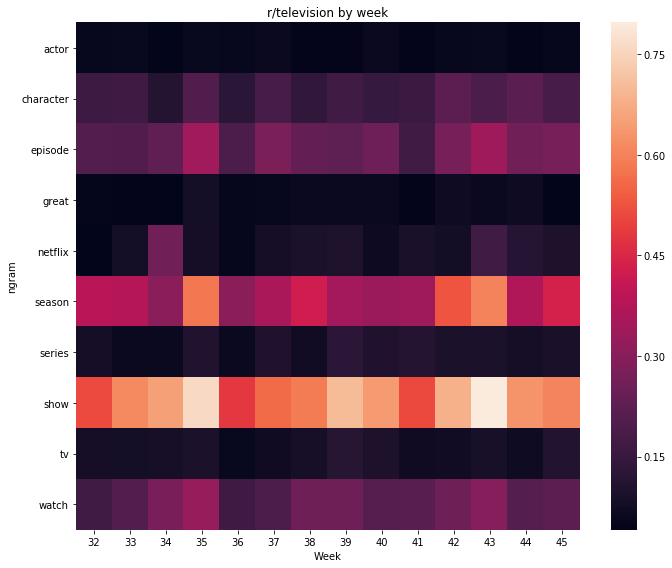
And it was quite boring, I’ve decided to try weekday heatmap, but it wasn’t
better as topics were the same:
weekday_heatmap(r_television_by_day, 'r/television weekday') # in the gist
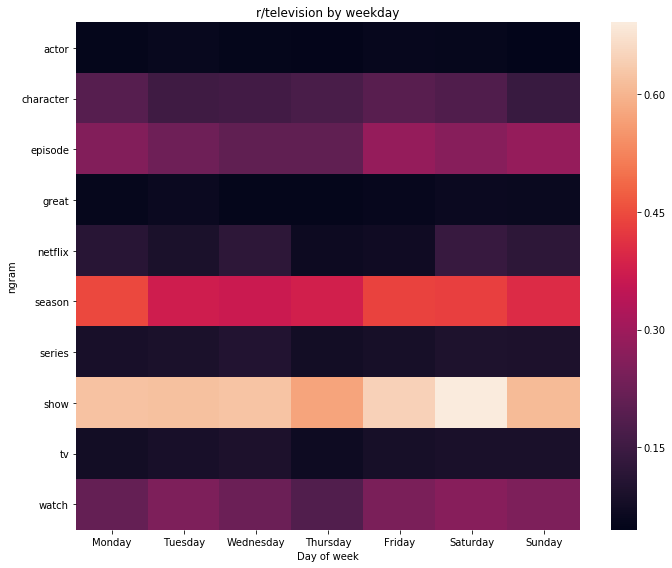
Heatmaps for r/programming are also boring:
r_programming_by_day = diff_n_by_day( # in the gist
50, whole_askreddit_df, 'programming', '2018-08-01', '2018-10-31',
exclude=['gt', 'use', 'write'], # selected manully
)
weekly_heatmap(r_programming_by_day, 'r/programming')
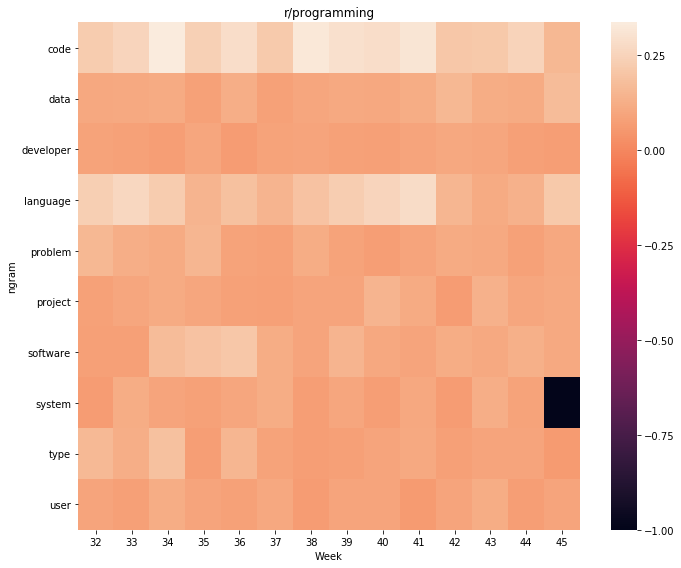
Although a heatmap by a weekday is a bit different:
weekday_heatmap(r_programming_by_day, 'r/programming by weekday')
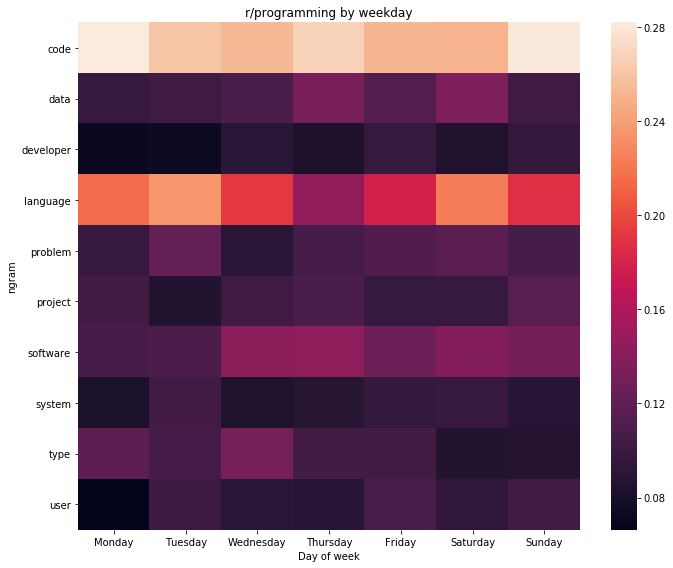
Another popular subreddit – r/sports:
r_sports_by_day = diff_n_by_day(
50, whole_askreddit_df, 'sports', '2018-08-01', '2018-10-31',
exclude=['r/sports'],
)
weekly_heatmap(r_sports_by_day, 'r/sports')
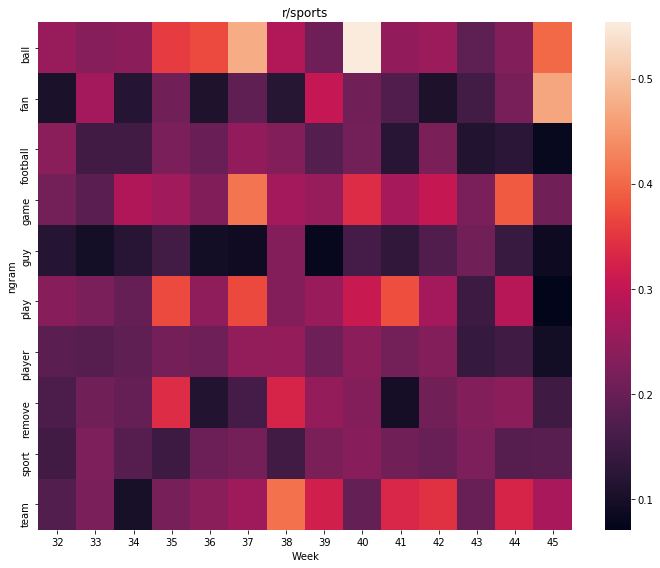
weekday_heatmap(r_sports_by_day, 'r/sports by weekday')
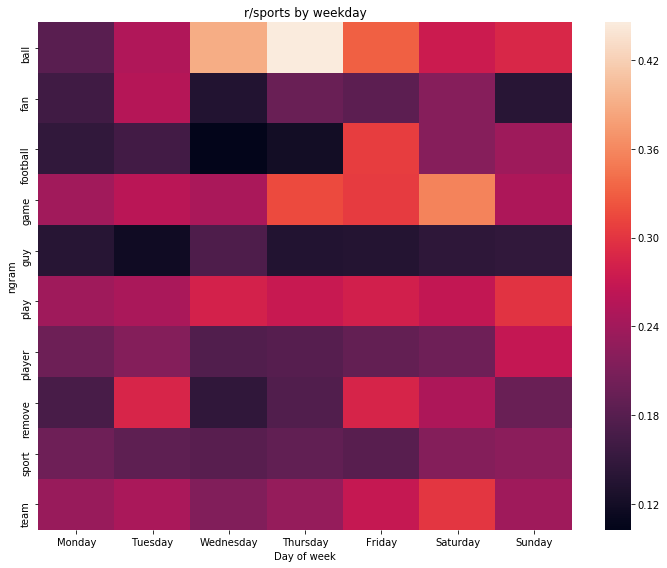
As the last subreddit for giggles – r/drunk:
r_drunk_by_day = diff_n_by_day(50, whole_askreddit_df, 'drunk', '2018-08-01', '2018-10-31')
weekly_heatmap(r_drunk_by_day, 'r/drunk')
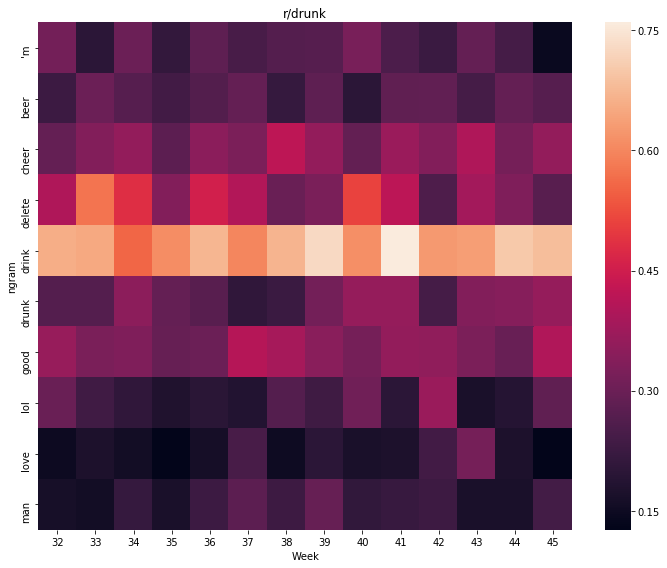
weekday_heatmap(r_drunk_by_day, "r/drunk by weekday")
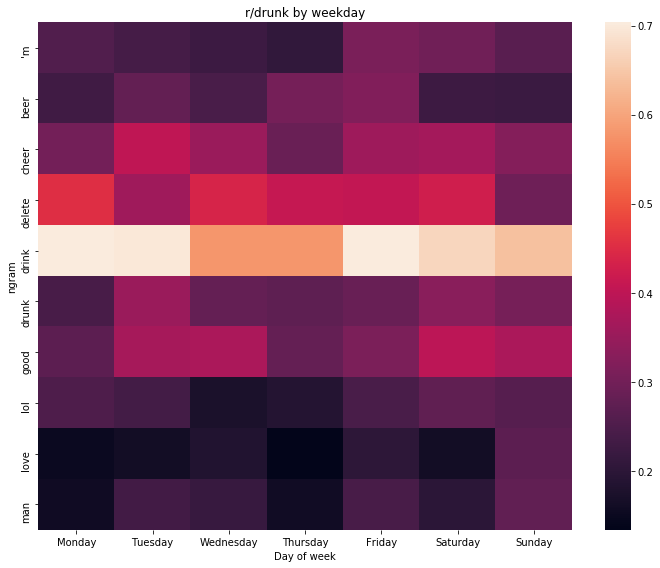
Conclusion
The idea kind of works for generic topics of subreddits, but can’t
be used for finding trends.
Gist with everything.